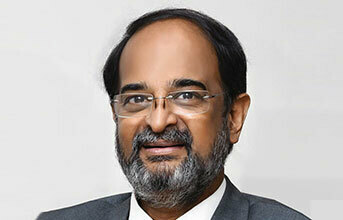
How has the adoption of big data analytics helped in transforming decision-making processes within the railway industry?
Indian Railways is the fourth largest in the world - it is an economic lifeline connecting the entire length and breadth of the country. While the sector has historically relied on legacy systems to maintain operations, there is a swift, discerning shift towards digitalisation. The Indian Railways has embraced digitalisation across spheres like operations, maintenance, and monitoring to enhance passenger experience and improve revenue.
The adoption of big data analytics has fundamentally reshaped decision-making within the railway sector. First, there's the modernisation of the signalling system designed to improve safety and line capacity. Second, we're able to monitor data using sensors in real-time, and identify and prevent issues - shifting from reactive to predictive maintenance. This is improving fleet reliability and availability, an important factor that influences revenue for railways. Lastly, big data is strengthening giant public corporations such as Indian Railways to maintain reliable schedules using real-time data, ultimately enhancing the experience, not just for passengers but also for all stakeholders.
How is Bahwan CyberTek (BCT) leveraging new technologies and methodologies used in big data analytics to reshape railway operations?
At BCT, we use our unique combination of IP products, services, and outcome-based business approach to deliver success to our clients. For the railways, specifically, we use our digital enterprise asset management (EAM) solutions to improve sustainability, safety, and cost in asset-intensive industries. Another important aspect of our offering is our proven and successful deployment of IBM Maximo, which is a leading asset management solution in the rail industry. BCT's knowledge capital, deep-domain expertise, and award-winning service capabilities perfectly complement the IBM Maximo solution, making us an ideal transformation partner.
BCT is one of the top 10 Global System Integrators for IBM Maximo. It has been transforming the infrastructure, transportation, roadways and railroad industries across India, The Middle East and far East, for over 15 years through integrated digital solutions for asset management, predictive maintenance, logistics and supply chain management. We've also partnered with the Mumbai Metro Rail Corporation Limited (MMRCL) in their pursuit to build a Common Assets Management System to optimise processes, perform lifecycle cost monitoring and improve efficiencies.
We understand that railway assets are complex. There are numerous coaches, several kilometres of tracks, unmanned territories, and many human operators at various points. We leverage the power of AI and IoT for data-driven decision-making, thereby helping railways move from condition-based maintenance to predictive maintenance and convert siloed asset management approaches into a single integrated approach for easy collaboration and decision-making.
How big data analytics has improved maintenance schedules and equipment failure prediction in the railway industry. Elucidate with a detailed case study?
Let me give you the example of the work we did for the Mumbai Metro Rail Corporation Limited (MMRCL). BCT was awarded a contract to build the Common Asset Management System (CAMS) for Mumbai Metro Line-3 (MML-3), by MMRCL, the nodal agency responsible for the implementation of the MML3 project in India. BCT was engaged to provide the end-to-end design, implementation, and support for CAMS, leveraging its expertise in delivering enterprise class EAM solutions.
With our dedicated team of technology experts, enterprise solution architects, and integration and quality assurance experts, we built an integrated CAMS to optimise processes, perform lifecycle cost monitoring and improve cost efficiency of MMRCL.
The CAMS solution helped MMRCL manage different types of assets such as rolling stock, tracks, power supply systems, traction systems, signalling and control systems, platform screen doors, telecommunication systems, plants and equipment, automated fare collection systems, escalators, and lifts, tunnel ventilation systems, security systems, infrastructure, and related systems and electro-mechanical systems like fire alarms, domestic water, lightning, etc. through a single window enterprise application.
How is Bahwan CyberTek leveraging big data in real-time monitoring of tracks, signals, and infrastructure to prevent accidents and unforeseen collisions?
Our comprehensive product and service offerings cater to the specific needs of the transportation industry, emphasising railway safety and efficiency. We can leverage big data analytics, IoT, AI, and ML driven predictive algorithms that gather data including the status and performance of equipment in real time and develop predictive models. This will also include data from sensors placed on tracks, signals, and trains, as well as external factors like weather conditions and historical accident data. These predictive models are designed to identify performance challenges, potential risks, equipment failures, and disruptive patterns. By processing vast datasets, we will be able to predict and enhance decision-making.
Using emerging technologies, BCT can assist railways in making informed, data-driven decisions. These decisions optimise resource allocation, streamline train scheduling, and improve route design, ultimately enhancing operational efficiency, sustainability, and safety.
Continue reading on Page 2